Current Projects
Using cognitive neuroscience to understand learning mechanisms: Evidence from phonological processing
Artificial grammar learning (AGL) studies have been widely used for testing the learnability of phonological patterns. It has been shown at the behavioral level that learners can learn and extract adjacent and non-adjacent dependencies with relatively short training. Less is known about how lab-learned patterns are encoded at the neurophysiological level. The aim of this project is to examine the neurophysiological correlates of different learning mechanisms when learning non-adjacent phonotactic patterns. We believe that understanding phonological processing will illuminate the learning mechanisms (domain-specific vs. domain-general) used to acquire language.
Using both behavioral and EEG/ERP measures, we are interested in the following questions:
Do domain-specific (linguistic) vs. domain-general mechanisms support learning new phonological patterns?
Are there reliable neurophysiological correlates of processing sound patterns?
Do different learning mechanisms lead to different types of neural observations?
In a behavioral experiment, we showed that when phonological patterns fall within the scope of human languages, they can be learned. When they fall outside, they are much harder to learn. This is a piece of direct evidence that the domain-specific phonological learning mechanism is limited by linguistic constraints.
We also look for neurophysiological correlates of phonological computation that can be detected during word processing using EEG. We find that the P3 component – reflecting categorization of phonotactically well-formed vs. ill-formed words – shows how fast the brain computes the phonotactic difference between words following the pattern and words violating it. In addition to the P3, we find a Late Positive Component (LPC) reflecting violations of non-adjacent phonotactic constraints that influence later stages of cognitive processing.
We also compare implicit (domain-specific) vs. explicit (domain-general) learning strategies. Implicit learning is how we learn our first language – it is cue-based, effortless, unconscious, and required no feedback. Explicit learning is how we often learn second languages – it is rule-based, effortful, conscious, and requires feedback. Our results show that explicit learning works – our participants showed high behavioral sensitivity to the pattern. However, while implicit learning leads to a measurable neural learning response, explicit learning leaves the brain silent.
Embedding phonological processing within cognitive neuroscience can reveal new insights into critical learning related questions. Our observations about learning and computing phonological non-adjacent dependencies suggest a complex interplay between domain-general and domain-specific learning and processing mechanisms.
ELAN, Index of a Failed Syntactic Prediction, in Garden-Path Sentences
In collaboration with Ryan Rhodes, we assume that ELAN, which is an early (150-200ms) brain response to unexpected or ungrammatical syntactic categories, should be elicited as an index failed syntactic prediction in garden-path sentences. We hypothesize that when the parser encounters a garden path, it makes a commitment to a particular syntactic structure. If that structure ultimately turns out to be incorrect, the parser will have to revise the parse when it reaches a word that violates its expectations. Thus, the disambiguating word in a garden path construction (assuming the parser has chosen and is committed to the wrong parse) should elicit an ELAN along with a late positive response (P600).
Subregular Complexity and Machine Learning
In collaboration with Dr. Shibata and Dr. Heinz, we are proposing that formal language theory provides a systematic way to better understand the kinds of patterns that neural network algorithms are able to learn. With this project, we are trying to get a glimpse of the black box problem of deep learning networks by systematically analyzing regular languages of which grammar is already known. Our aim is to simulate the underlying grammars that the machines extracted from the training data. We also compare the RNNs (with LSTM) and RPNI algorithms which outputs a DFA (by state merging) that is always consistent with the learning data (de la Higuera, C., 2010) in order to see whether RNNs need more or less training data than RPNI.
Past Projects
Mirror Frequencies in Early Child Lexical Development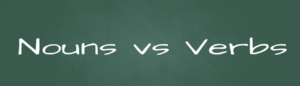
In collaboration with Dr. Cem Bozsahin, we were investigating the nature of early lexical acquisition in Turkish. We hypothesized that most frequent words in child-directed speech should be acquired first, provided they are also less ambiguous in terms of argument specificity, and morphologically less complex than other exposures in the same time frame, independent of their lexical class. We observed that frequency analyses of child-speech and child-directed speech lend support to a mechanistic explanation of early lexical development in the manner above, rather than cognitivist explanations (see Bozsahin 2012, Clark and Lappin 2013 for some discussion). We think that the nouns-first claim (Genter, 1982), and the counterclaims based on cultural biases (Brown, 1998; Tardif, 1996), need rethinking in non-cognitivist terms, and more in empirical/computationalist/localist terms, where the kind of behavior in child-directed speech (CDS) and child speech (CS) correlate. We report Turkish data to support our claim, where such correspondence is made evident in surface morphology (Xanthos et al. 2011).
Sensitivity of Turkish infants to vowel harmony: Preference shift from familiarity to novelty
This is research was a collaborative work of METU BeBeM Laboratory which is directed by Dr. Annette Hohenberger. In this study, using the head-turn paradigm, we found that 6- and 10-month-old monolingual Turkish infants are already sensitive to backness vowel harmony in stem-suffix sequences. It was concluded that this finding is reminiscent of the “familiarity-to-novelty-shift” in cognitive development, indicating that younger infants first extract the regular, harmonic pattern in their ambient language, whereas older infants’ attention is drawn to irregular, disharmonic tokens, due to violation-of-expectation.